Keywords
Clinical knowledge, clinical reasoning skills, problem-solving prior to instruction, situated learning, transfer
Introduction
A major problem in medical education is that the clinical knowledge and clinical reasoning skills acquired through university teaching do not transfer well to clinical practice. Despite acquiring massive amounts of content knowledge about the functioning of the human body, medical students struggle to transfer that knowledge to one of the core disciplinary practices – differential diagnosis. Related to differential diagnosis are (a) clinical knowledge which describes declarative/conceptual knowledge about specific diseases and the process of differential diagnosis and (b) clinical reasoning skills which describe strategic knowledge in the decision-making process associated with differential diagnosis. The lack to transfer may stem from current methods of instruction which are focused primarily on imparting massive amounts of basic content knowledge without adequate attention to situate this knowledge in disciplinary practice (Collart et al., 2016, Norman, 2009). A possible solution to this problem is to expose and link the learning of medical students to the practice of differential diagnosis. This approach is supported by theories of situated cognition. Whilst we acknowledge that there are several options to integrate situated learning, we aim to explore the use of medical computer-based virtual environment (CVE) simulations. In empirical research it has yet to be shown why and when CVE simulations are effective in medical education and enhancing transfer. Inter alia, the ability to transfer is important because medical students cannot be confronted with all possible situations in their medical studies they will face later in their professional career as doctors. We annotate that in this study we focused clinical knowledge and the conceptual aspects of differential diagnosis and not on the execution of procedures. Please refer to figure 1 or the proposed project approach.

Figure 1. Proposed Project Approach. Abbreviations: CK: clinical knowledge; CRS: clinical reasoning
Goals
The first major goal of this thesis was to overcome the issue of transfer in differential diagnosis by using a situated learning approach. Furthermore, we pursued the second major goal of evaluation how, when, and why CVE simulations are effective in medical education. We aimed to achieve these goals by carrying out the following three tasks, split up into three work packages.
- Examine the effect of problem-solving in CVEs on clinical knowledge and clinical reasoning skills isomorphic testing and transfer outcomes and evoked learning mechanisms when combined with direct video instruction in different sequences.
- Extend the research on the problem-solving prior to instruction approach by altering the timing of provided feedback during the problem-solving phase and enhance the preparatory effects during CVE problem-solving for future learning.
- Compare the influence of semester-long exposure to problem-solving in CVE preceding direct instruction to group discussions preceding the lecture.
Furthermore, findings of the present project might provide an explanatory basis for informed decision making about the future application of CVE learning platforms in the medical trajectory at ETH Zürich. Please refer to Figure 2 for an illustration of the implemented CVE platform.

Figure 2. Implemented CVE Platform. Figure reprinted with permission of I-Human Patients by Kaplan, https://www.i-human.com.
State of the project
In the first study we used a problem-solving prior to direct instruction (Sinha & Kapur, 2021, Loibl et al., 2017) versus instruction first experimental design. We compared the effect of problem-solving in CVEs prior and after direct instruction on isomorphic testing and transfer outcomes by assessing declarative and conceptual clinical knowledge and clinical reasoning skills as well as with evoked learning mechanisms. The study took place in spring 2021 with undergraduate medical students in the scope of a medical trajectory course. Due to Corona-Virus-19 issues, all study activities in this thesis were conducted remotely where participants worked individually from home. We did not find any learning activity sequence to be superior to the other. However, when looking at the two learning activities individually, they found that problem-solving in CVE simulations and direct instruction might be equally effective for learning content knowledge. Nevertheless, problem-solving in CVEs with formative feedback might be more effective for learning clinical reasoning skills better than mere instruction.
In the second study we extended the research on the problem-solving prior to instruction approach and put the findings of study 1 into practice to further enhance the acquisition and transfer of clinical knowledge and clinical reasoning skills through problem-solving in CVE prior to instruction. We did so by altering the timing of provided formative feedback during the CVE problem-solving phase. This was represented by instant, delayed, and no feedback provision. Again, we evaluated triggered learning mechanism. The study took place in spring 2022 with undergraduate medical students in the scope of a medical trajectory course. Due to Corona-Virus-19 issues, all study activities in this thesis were conducted remotely where participants worked individually from home. The findings of this study revealed that all three timings of investigated feedback might be eligible because none of them had detrimental effects on learning. However, if and at what point in time feedback should be provided to optimally enhance learning, heavily depends on the targeted learning outcome, which might be clinical declarative or conceptual knowledge, clinical reasoning skills, and respective acquisition or transfer.
In the third cohort-based study, we explored the influence of semester-long exposure to problem-solving in CVE prior to introductory lectures versus interactive group discussions preceding the lectures in an introductory course on performance in a successive advanced course in the medical trajectory, both related to differential diagnosis. Using a double transfer experimental design, we compared the effects of the two teaching approaches on acquisition of clinical knowledge, transfer of clinical reasoning skills, and students’ satisfaction and self-confidence in learning. The study took place in spring semester 2021 and 2022 with undergraduate medical students in the scope of a medical trajectory course. Due to Corona-Virus-19 issues, all study activities in this thesis were conducted remotely where participants worked individually from home. We found both group discussion and CVE problem-solving preceding an instructional lecture to prepare students for the acquisition of declarative and conceptual clinical knowledge in an advanced differential diagnosis course. However, the group discussions preceding the lecture might be more effective than CVE problem-solving preceding the lecture. Furthermore, we found interactive group discussions preceding the lecture in the basic differential diagnosis course to be more effective in enhancing clinical reasoning skills transfer, but only when the transfer problem featured specific configurations. However, we did not find a difference in satisfaction and self-confidence in learning with simulations between cohorts nor any mediating effect of simulation design features on learning outcomes. However, CVE problem-solving preceding the instructional lecture was equally effective than group discussions in (a) enhancing transfer of clinical reasoning skills when specific testing configurations were met and (b) preparing students for the acquisition of declarative and conceptual clinical knowledge. Consequently, from a practical side of view CVE problem-solving seems to be a plausible alternative to group discussions as preparatory activity for following instruction.
References
Collard, Anne & Bredart, Serge & Bourguignon, J.-P. (2015). Context impact of clinical scenario on knowledge transfer and reasoning capacity in a medical problem-based learning curriculum. Higher Education Research & Development, 35(2), 242-253. https://doi.org/10.1080/07294360.2015.1087383
Loibl, K., Roll, I., & Rummel, N. (2017). Towards a Theory of When and How Problem Solving Followed by Instruction Supports Learning. Educational Psychology Review, 29(4), 693–715. https://doi.org/10.1007/s10648-016-9379-x
Norman, G. (2009). Teaching basic science to optimize transfer. Medical Teacher, 31(9), 807–811. https://doi.org/10.1080/01421590903049814
Sinha, T., & Kapur, M. (2021). When Problem Solving Followed by Instruction Works: Evidence for Productive Failure. Review of Educational Research, 91(5), 761–798. https://doi.org/10.3102/00346543211019105
Publications
Poster presentations:
Fässler, C. (2021, August). Computer-Based Virtual Environment Simulations for Differential Diagnosis in Medical Education. Poster presented at the 25th meeting of the JURE Pre-Conference for Research on Learning and Instruction, Online.
Fässler, C. (2021, September). Sequencing of Problem-Solving in CVE Scenarios and Direct Video Instruction. Poster presented at the 17th annual meeting of the GMA (Gemeinschaft für Medizinische Ausbildung), Online.
Articles:
Fässler C, Sinha T, Schmied CM et al. Problem-solving in virtual environment simulations prior to direct instruction for differential diagnosis in medical education: An experimental study [version 2; peer review: 2 approved with reservations]. MedEdPublish 2023, 12:61. https://doi.org/10.12688/mep.1

Dr. Christian Fässler
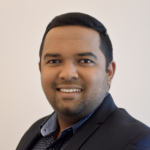
Dr. Tanmay Sinha
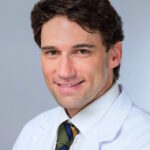
PD Dr. Christian Schmied

Prof. Dr. Elena Osto
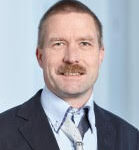
Prof. Dr. Jörg Goldhahn
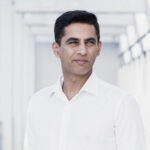