Keywords
Intuitive physics, misconceptions, neuroimaging, fMRI
Introduction
We successfully interact with the physics of the world every day. Having an intuitive understanding of the environment’s physical properties and dynamics allows us to predict the behaviour of objects, for example, when they fall, slide, or collide. It also allows us to deliberately act upon them, by applying the right forces to produce an intended outcome. Neuroscience research suggests that this ability relies on internal models of the physical world and reflects Bayesian inference (for a review, see Kubricht, Holyoak & Lu, 2017). The most prevalent theoretical approach to intuitive physics is the noisy Newton framework, which suggests that people’s physical judgments correspond to optimal probabilistic inference over a Newtonian physical model that incorporates uncertainty about the sensory inputs (Jörges & López-Moliner, 2017; Sanborn, Mansinghka & Griffiths, 2009; Sanborn, Mansinghka & Griffiths, 2013; Smith & Vul, 2013). In other words, models based on this framework assume that when exposed to a physical situation, people’s prior beliefs about the physical and perceptual variables at play are integrated with noisy sensory information, in accordance with ground-truth physical principles.
In contrast, despite being very adept at operating in the physical world, we are typically poor at describing or explicitly reasoning about it. This is illustrated by common misconceptions that people exhibit when solving seemingly simple physics problems (Kubricht, 2017). For example, when asked to estimate the trajectory of an object falling from a moving body, people often mistakenly predict it to fall straight-down (McCloskey & al., 1983).
The noisy Newton framework has been successful at modelling human performance for a variety of physical tasks, such as mass judgment (Sanborn, Mansinghka & Griffiths, 2009) or ballistic motion estimation (Smith, Battaglia & Vul, 2013). However, this approach alone cannot account for people’s systematic (i.e., non-random) errors in judgments reflected in misconceptions, because the physical properties and dynamics approximated by the model are biased by noise rather than structured deviations. It may be that in some cases, our inferences rely on prior assumptions that are embedded in erroneous physical models that may reflect heuristics.
More specifically, we propose that when making physical judgments, people draw on a set of prior assumptions that have been acquired through active experimentation when solving physical problems either explicitly or via interactions with the world. However, which prior guides a person’s inference might be influenced by an “availability heuristic”, i.e. a mental shortcut that relies on examples that come to mind when evaluating a specific problem. This would predict that misconceptions result from relying on priors which are incorrect but easy to retrieve from memory, for example, because they have been experienced frequently or recently.
Goals
The aim of this project is to gain a better understanding of how the brain performs physical inference, by investigating the influence of contextual factors on (i) whether the inference is erroneous, (ii) how an erroneous conception can be corrected, and (iii) which neural mechanisms underpin these processes. This will be achieved by using a virtual physical world to measure potential misconceptions, examine how physical inference is influenced by contextual information, and what neural mechanisms support physical inference. This will provide a window into how the brain learns about the properties and dynamics of the physical environment, and might open new avenues for targeting physics misconceptions in learning sciences.
State of the project
To study intuitive physical inference, we created a dynamical 3D physics world and asked healthy young adults to solve a simple physics task which, when presented in paper-and-pencil format, typically reveals a “straight-down” misconception. This misconception corresponds to the erroneous belief that an object falling from a moving body will fall straight-down.
In order to solve the computer-based physics task, participants have to estimate the landing position of a ball falling from a moving object, without seeing the trajectory the ball. During the instructional phase of the experiment, participants get familiarized with the effects of gravity in the 3D world by viewing a series of falling balls.
In a behavioural experiment, we investigated whether the likelihood of exhibiting a straight-down misconception is influenced by immediate prior experience. To do so, we manipulated the instructions such that one group only saw a ball falling straight-down from a static object, while another group saw balls arriving from outside of the bounds of the screen and falling in parabolic motion. Both scenes followed Newtonian physics, and none involved a moving object. Our hypothesis was that recent exposure to parabolic motion would decrease the likelihood of exhibiting a straight-down misconception.
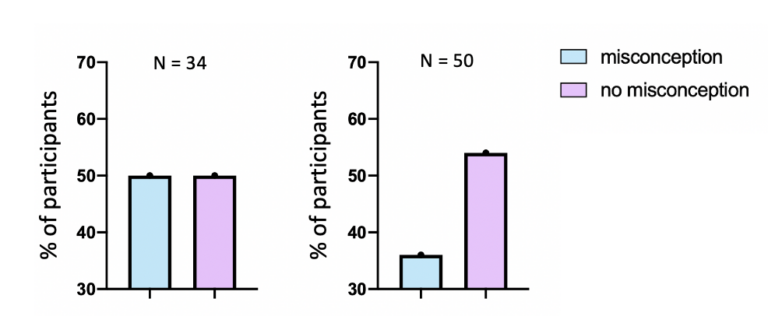
Figure 1. Preliminary results of the behavioural experiment. (A) 50% of the 34 participants previously exposed to a ball falling straight-down exhibited a misconception (B) 36% of the 50 participants previously exposed to balls falling in parabolic motion exhibited a straight-down misconception.
Preliminary results showed that 50% of the participants exhibited a straight-down misconception in the group previously exposed to a ball falling straight-down, and 36% in the group previously exposed to parabolic motion (see Figure 1). This difference could be explained by a potential « availability heuristic » influencing the misconception. However, a larger sample size is needed to draw firm conclusions. For this reason, we are developing a web-based experiment.
To investigate the neural bases of intuitive physical inference, we translated our behavioural paradigm into a functional neuroimaging (fMRI) experiment. The fMRI experiment was preceded by a training session during which participants performed the computer task while receiving feedback on their performance.
Preliminary results showed that performing the physics task (without seeing the falling ball), in comparison to performing an attentional task involving the same visual stimuli but not requiring physical inference, activated motor and parietal regions, which have previously been shown to be involved in intuitive physical inference (Fischer & al., 2016), MTV5, which is responsible for motion perception, and the insula (see Figure 2).
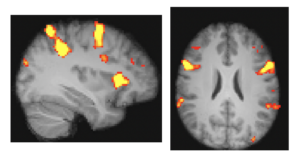
Figure 2. Preliminary group fMRI results of 4 participants, for the physics task > control task contrast.
References
Fischer, J., Mikhael, J. G., Tenenbaum, J. B., & Kanwisher, N. (2016). Functional neuroanatomy of intuitive physical inference. Proceedings of the national academy of sciences, 113(34), E5072-E5081.
Jörges, B., & López-Moliner, J. (2017). Gravity as a strong prior: implications for perception and action. Frontiers in human neuroscience, 11, 203.
McCloskey, M., Caramazza, A., & Green, B. (1980). Curvilinear motion in the absence of external forces: Naive beliefs about the motion of objects. Science, 210(4474), 1139-1141.
McCloskey, M., Washburn, A., & Felch, L. (1983). Intuitive physics: the straight-down belief and its origin. Journal of Experimental Psychology: Learning, Memory, and Cognition, 9(4), 636.
Sanborn, A. N., Mansinghka, V. K., & Griffiths, T. L. (2009). A Bayesian framework for modeling intuitive dynamics. In Proceedings of the 31st annual conference of the cognitive science society (pp. 1-6).
Sanborn, A. N., Mansinghka, V. K., & Griffiths, T. L. (2013). Reconciling intuitive physics and Newtonian mechanics for colliding objects. Psychological review, 120(2), 411.
Smith, K. A., Battaglia, P., & Vul, E. (2013). Consistent physics underlying ballistic motion prediction. In Proceedings of the Annual Meeting of the Cognitive Science Society (Vol. 35, No. 35).
Project team
Alumni
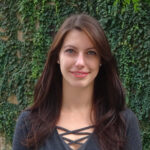